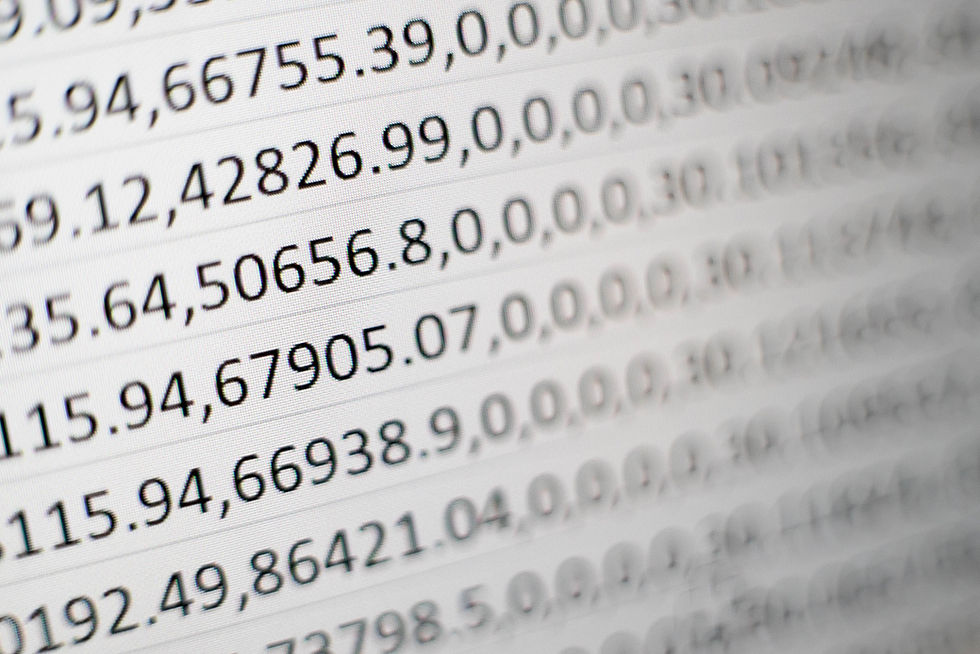
We keep hearing of "big data" but in reality, a lot of businesses don't have the overwhelming amounts of data that industry experts say is available. For many businesses, the types and quantity of data that is readily available hasn't changed all that much over the last 10 years. Sure the business could buy additional data (at a cost) but most businesses will have their own ex-DC data, and for a segment of their range may have some scan or sell-through data. There will be some pricing information and maybe some other marketing and sales data but overall it may be a bit sketchy.
But there may be data that you can use and it comes in different forms. These can be used to improve your market understanding and improve accuracy.
The first type of data is the characteristics of your existing historical demand and this can be used to develop a powerful forecast. On top of seasonality and trend there is a myriad other factors such as a recurring yearly profile, a long term business cycle, or a daily purchasing profile. Traditional demand planning tools often overlook these because of the algorithms they use. By using this information you will have a more information-rich forecast compared to the common old-style methodologies.
It doesn't end there. Importantly with a flexible forecasting algorithm you can easily build on this by adding the data that you have, such as price, to further improve accuracy. And a good solution will help you to understand the quality of the data and improve it when developing the models - a more agile approach.
You can achieve additional improvements by using data that is freely available. Something as simple as the number of school days per week or when public holidays occur can improve accuracy. There may be information available from government and industry bodies such as housing approvals, tourist numbers and weather information. With Cauself we are making it easier for companies to collect and use this information.
Once these causal drivers have been collected then there are variations of them that could be used to deliver further benefits. For example, if you sold building products you probably see a delayed effect from housing approvals and this can be reflected by having a causal offset. For a product that is affected by temperature, rather than a weekly average temperature a better predictor may be a count of the number of days over 30 degrees. By taking an existing causal and aligning it to how consumers actually react can help to deliver insights and improve accuracy.
Once you have all this information then what do you with it?
A traditional demand planning solution may let you view the causal factors in the forecast screen, but they don't change how the forecasts are calculated.
This is where Cauself differs - the solution can analyse up to 20 different factors and then determine the ones that are the biggest drivers and use these to establish an advanced statistical model specific to that product. It could identify that baseline demand is driven by an economic factor like market sentiment, while an event like a promotion is price-driven.
It's these capabilities that provide you real insights and break down the barriers to improving accuracy.
Want to learn more - Contact Cauself.
Comentários