We have all heard about the "agile" approach - such as agile software development and agile implementation methodologies. Unfortunately it hasn't reached the world of data analytics and statistical modelling... until now.
Historically statistical modelling has been slow and clumsy, and data quality is often cited as the cause. The story goes something like this. Data is sent to a data scientist to create the models only to find that the data is missing or incorrect. This means that you need to go through a data cleansing process before the data scientist can do their thing. The corrected data is sent back to the data scientist and a few weeks later you get some results.
It shouldn't be that way.
By seeing how the statistical models react to your data as-is you can start to see where there may be data quality issues, and you can make amendments on the spot and see the effects immediately. For instance - you may identify that there is an unusual peak that the model didn't pick up on and this could be because a causal factor was incorrectly defined or missing. By correcting this you can see the immediate effect of that missing value.
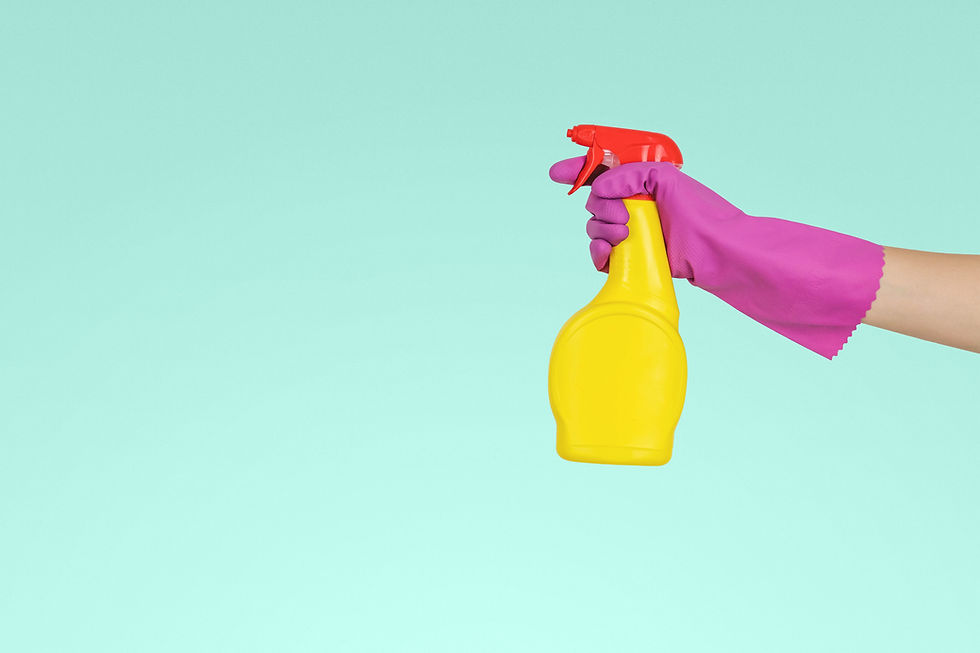
By adopting an agile approach you will get results more quickly and by using the data that you have you will see powerful insights that will not only improve how you plan your business, but also make the data collection process more effective going forward.
Want to learn more - contact the team at Cauself.
Comments